Session Aims & Scope
The additive manufacturing (AM) process, such as LPBF, is a dynamic and transient process that is not in a steady state and lacks equilibrium. The formation of defects is often associated with intricate thermal phenomena that involve the melting, cooling, solidification, and re-melting of powders. The process parameters are typically determined through cycle testing, resulting in an open-loop manufacturing process that accumulates quality defects, thus posing significant challenges in process control. By integrating process mechanics and monitoring data using machine learning techniques, Digital Twin-based AM systems can model the cause-and-effect relationship between quality defects and process features, enabling real-time monitoring and control of the microstructure and build quality. The digital twins-based AM process modeling and control approaches, are expected to address the quality and repeatability issues in additive manufacturing processes.
Session focuses
The session will focus on the following points
- Physical modeling of AM process
- AM process monitoring and control
- Physics-informed process modeling of AM process
- Digital twin of AM system and it’s applications
Session Chair(s)
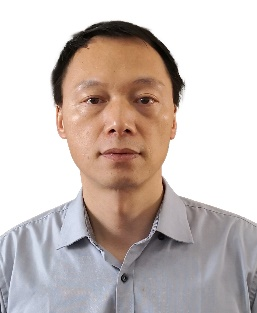
Chair
Kunpeng Zhu
Hefei Institutes of Physical Science, Chinese Academy of Sciences
Wuhan university of science and technology
zhukp@iamt.ac.cn
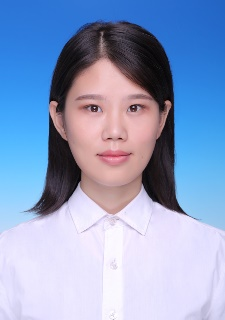
Co-Chair
Xin Lin
Wuhan university of science and technology
xinlin@wust.edu.cn
Session Presentation
1.
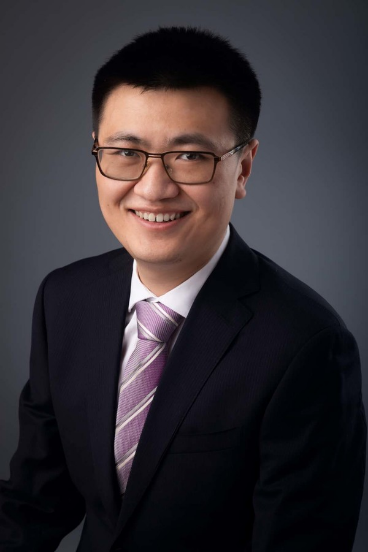
Yunlong Tang
Lecturer, Monash University (Australia)
Title: Service oriented digital twin for additive manufacturing process
Abstract
Currently, Industry 4.0 is rapidly developing, empowered by IoT, cloud computing and AI. As two crucial components of Industry 4.0, digital twins and additive manufacturing (AM) are attracting increasing attention. Despite considerable research in recent years on developing digital twins for AM, the field still faces discrepancies in definition and confusion in development processes, making the development of AM digital twins expensive. The reason behind this is the uniqueness of each AM process, leading to low adaptability in AM digital twins. To address this issue, this talk summarises AM digital twins found in the literature review and proposes a novel, service-oriented framework comprising four layers: service, model, data, and interface. This framework aims to enhance the reusability of developed AM digital twins across various levels. The commonly used components in each layer are also summarised to assist developers in rapidly constructing AM digital twins tailored to their specific needs. A case study is included to demonstrate the framework’s effectiveness and potential.
2.
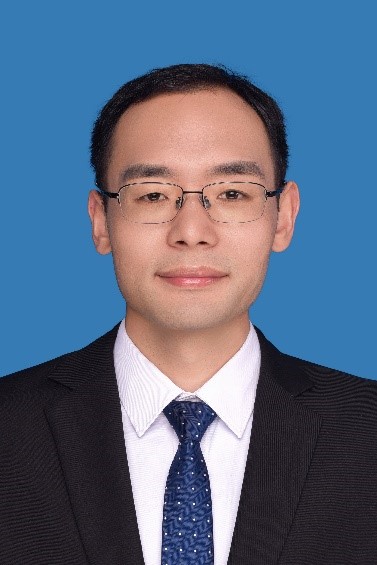
Xianyin Duan
Associate Professor, Wuhan University of Science and Technology (China)
Title: Digital Twin-driven On-line Monitoring Method of Laser Powder Bed Fusion Process
Abstract
The field of laser powder bed fusion (LPBF) of metals is advancing towards greater precision, efficiency, and intelligence. However, the extreme thermal conditions of the laser melting process have led to persistent quality defects in the fabricated parts, limiting the widespread application of LPBF technology. To address these challenges and produce high-quality parts, it is crucial to implement on-line monitoring and process control during manufacturing. This talk describes a digital twin-driven on-line monitoring method for the metal LPBF process. This method aims to establish an intelligent manufacturing system and perform case analysis. By integrating physical and virtual models of the powder melting process, on-line process monitoring, and big data analysis, a comprehensive digital twin system for the on-line monitoring of the building process is developed. This system encompasses the equipment layer, data conversion layer, network layer, control layer, and application layer. The talk details the functions and key technologies of each layer and provides analysis of typical application examples in selective laser melting (SLM) process monitoring. This approach offers theoretical and technical support for the intelligent manufacturing based on LPBF technology.
3.
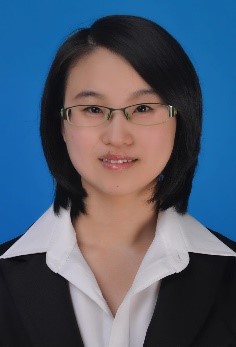
Yingjie Zhang
Associate Professor, South China University of Technology (China)
Title: A digital twin framework for melt pool monitoring of LPBF using machine learning with high-speed coaxial imaging and simulation data
Abstract
Digital twin technology is crucial for advancing intelligent manufacturing by ensuring product quality, reducing costs, and enhancing efficiency. In Laser Powder Bed Fusion (LPBF), Digital Twin technology provides a robust solution for predicting part characteristics, diagnosing defects, and controlling processes. This study introduces a sensor and simulation combined digital twin (SSC-DT) framework that monitors molten pool characteristics—such as width, depth, and mean temperature—by integrating in-situ sensor data with physical simulation data.
Experimental results show that the SSC-DT framework achieves a mean relative error of less than 10% compared to optical microscope (OM) measurements. Notably, the predicted molten pool depth error from SSC-DT is lower than those calculated by the Eagar-Tsai model, keyhole empirical model, and finite element method (FEM). Consequently, SSC-DT predictions closely align with actual sensor data under conduction and slight keyhole modes of the molten pool, supporting intelligent control, predictive maintenance, and quality assurance in future LPBF processes.